es, we know what Villanelle, one of the funniest killers ever appeared on the screen, would say about this article: "this is so boooooring!". We agree with Villanelle. Discussing Big Data sampling methodologies is not exactly the definition of sexy. But if you are currently relying on any kind of digital data providers for your insights, well this short (but boooooring) read is definitely worth your attention.
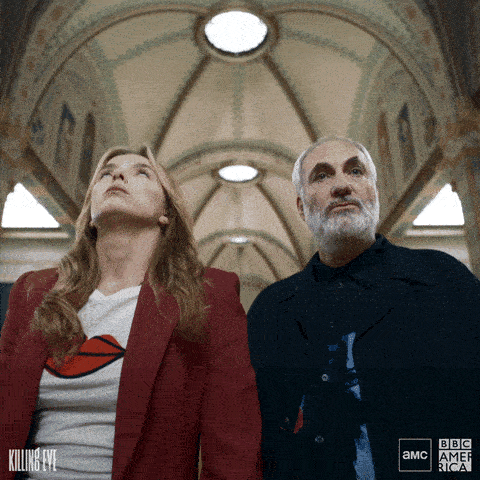
Raise your hand if you ever saw a Social Media data analysis where the presenter proudly declared "This study is based on millions of tweets and posts", as a proof of their analysis' solidity. This cheap trick was initially intended to shock marketers, used to the tiny numbers of traditional sampling. But with more than 500 millions tweets sent each day, a few millions data points is not meaningful at all - it's actually 100% unreliable. As live digital data sampling is actually impossible - as we will discover in a minute - the million tweets and posts boasted in these analysis turn to be the final nail in the coffin of their own reliability and credibility.
"Raise your hand if you ever saw a Social Media data analysis where the presenter proudly declared: "This study is based on millions of tweets and posts", as a proof of their analysis' solidity."
Sampling ≠ random extraction
As of 2020, 5,83 billion pages have been indexed on the visible part of the World Wide Web, almost doubling since 2012. In terms of content size, this means that it would currently take you 10 trillion years to download the whole Web from your computer.
So when it comes to digital data - and e-commerce data and consumer reviews in particular -Big Data is quite an understatement. To make things even more challenging, these data are growing at a staggering pace, continuously changing both in size and nature. This means that we can't apply sampling to digital data analytics, as we do in Market Research. Sampling is technically impossible here, because we do not know the size and the stratification of the universe we want to sample in the first place. Any "sampling" in this field would actually mean a meaningless random extraction, a.k.a. accidental sampling; the statistical equivalent of throwing a coin in the air. And yet this is what the vast majority of digital data analytics providers have done in the last ten years, especially in the field of Social Media Listening. Many DaaS companies have grown to the status of global data providers using big, random extractions of digital data, often shielding themselves behind the sheer size of the dataset they were pulling insights from.
"Any "sampling" in this field would actually mean a meaningless random extraction; the statistical equivalent of throwing a coin in the air."
To marketers used to the average sample size of traditional market research, a data provider claiming huge sample size might have initially sounded like an incredible endeavor ("Wow!!! Millions of data points!"). But a million data points is of no significance, if the size and quality of the universe is unknown and ever-changing. And in fact, these analysis have proven to be pretty useless and shallow. Frustration about many digital data platform - and Social Media Listening tools in particular - is a widespread phenomenon in the marketing community today.
Digital data are a huge, uncharted territory
With digital data analytics we are - quite literally - in uncharted territory. Using sampling techniques would be equal to infer that, just because there's white sand on the shore, we must have landed on a tropical island. But cartographers in the Middle Ages already knew that there's only one way to map out the nature of an unknown territory; to navigate the whole shoreline and chart the whole map.
Similarly, in digital data analytics we have only one chance to provide statistically valid datasets and insights: we have to take a complete census of the universe we want to analyze. There are no shortcuts, there is no mathematical magic that we can use to avoid this simple fact. If we want to know how is the sentiment on Twitter in China in 2019, we need to analyze every single tweet. And if we want to know if a product that gets a 4.1 rating on Amazon is really competitive, we need to analyze the performance of every single product sold on Amazon in that same category.
"In Big Data there's no such a thing as marginal data."
Because taking a complete data census is a scary task, many limit the analysis to just a few Brands or key topics. But that can't work either. Focusing only on main competitors or market leaders alone is a sure recipe for disaster. One might think that minor Brands are marginal data, that have only a small impact in terms of market influence, but in Big Data there's no such a thing as marginal data. We're living times in which categories are flooded with new Brands and products literally every day. Many start-ups or co-packers are trying their fortune fast-prototyping new concepts. Only a few might succeed, but the collective impact of these small initiatives is very significative. Analyzing only a few big Brands implies missing the emerging trends completely, only to identify them when it's too late.
Let's have a look at what happens in the Diapers market in China. Every Chinese co-packer is trying to get a larger slice of the pice by launching several new Brand concepts every quarter. In Q2-20 only, the number of Brands sold in the category grew up to 319, a 10% increase. In the Wipes market, the increase was a whopping +35% up to the current 411 Brands.
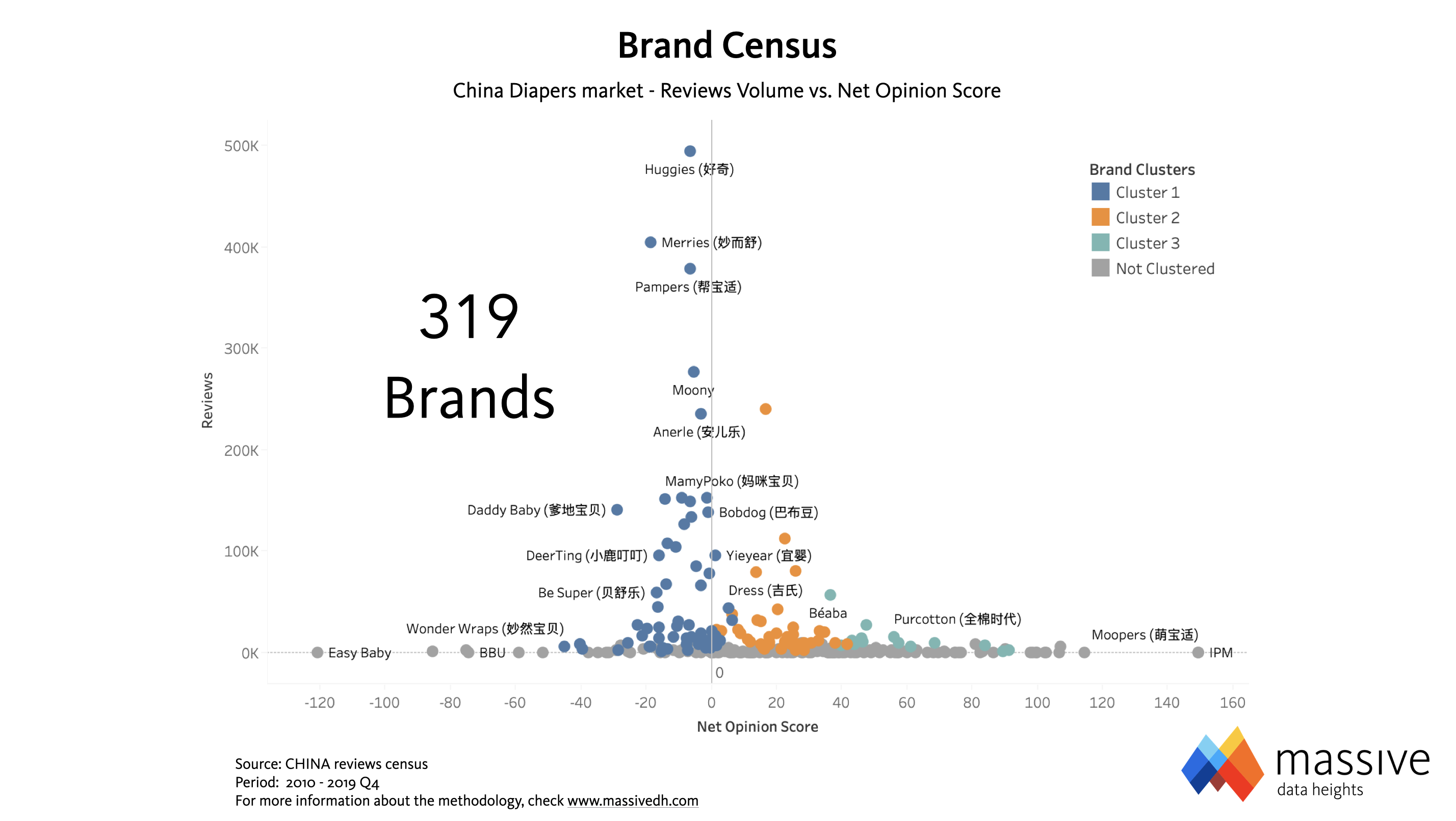
Many markets look like this today. Think of Beverages, or Cosmetics; no industry is immune in the start-up mindset era. In the image above, Cluster 1 (blue dots) is represented by the biggest 65, incumbent diapers Brands in China. They make the majority of volumes, but they show a negative sentiment (Net Opinion Score) overall, as they all sit on the left of the vertical axis. Clusters 2 and 3 (orange and light green dots respectively) only count 55 Brands combined, but their general sentiment is very positive as they make their consumers happier. These are all small Brands that did not exist a few years ago, collectively shifting the market in their favor and driving the trends. And their influence keeps growing, as they have gained 10 share points YoY reaching one third of the reviews volume now (image below: reviews share is a very close proxy of online sales share).
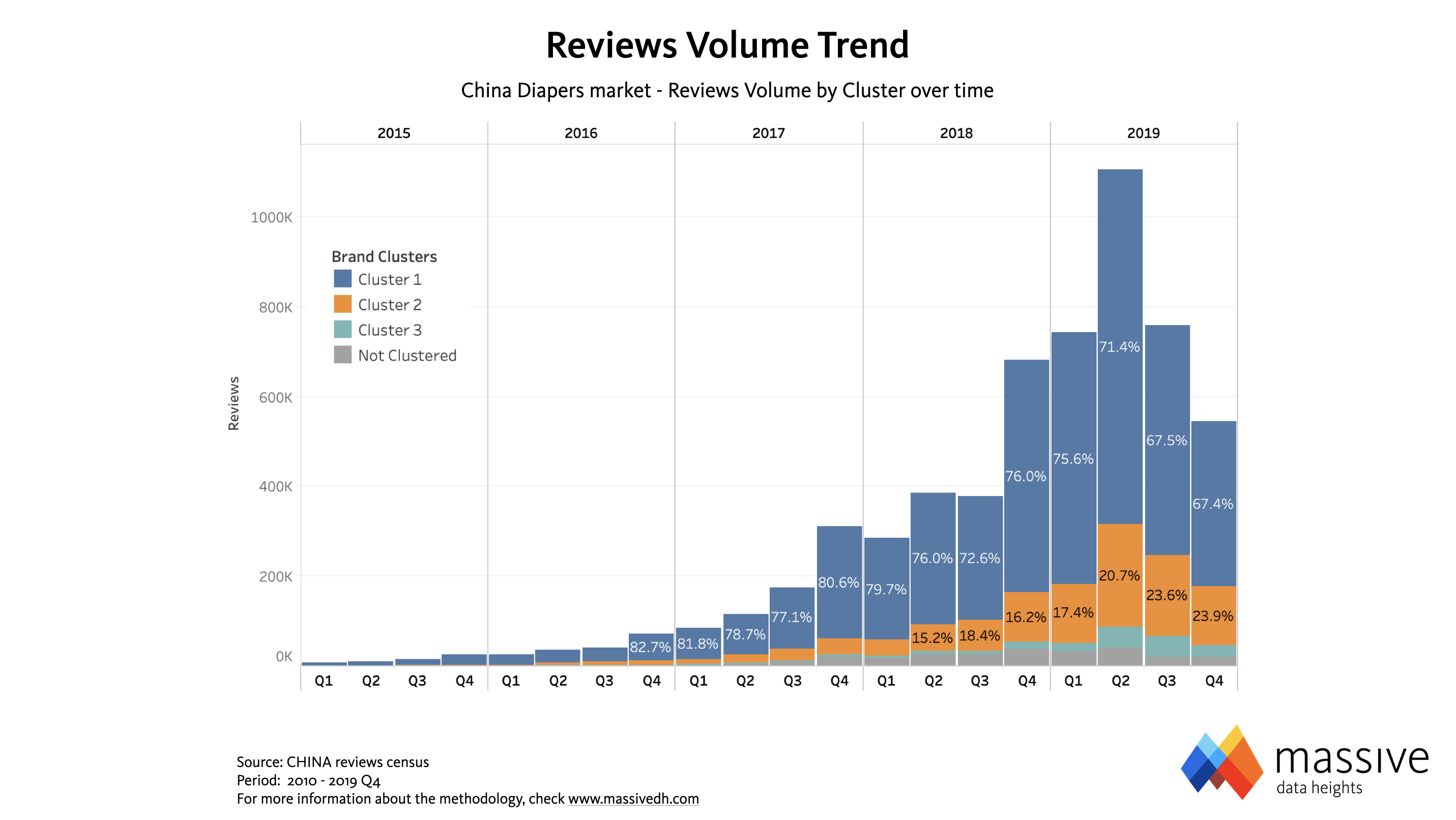
The analysis above clearly shows that to understand competitive dynamics, the market must be analyzed as a whole. The classic Market Research approach to run - i.e. tu run the study only on a smaller selection of competitors - was justified by traditional tools' impossibility to analyze the whole market. Back then, selection bias was a necessary evil caused by the limits of the technology available. But with today's Artificial Intelligence capabilities, using the same approach is just plain wrong.
So if you're on the market looking for a digital data provider, ask this simple question: are you sampling your datasets or do you take a complete census? Now you know the answer you want to hear.